Article Title :
Terrain Variables Based Spatial Mapping of Soil Properties in a Watershed of Himalayan Landscape Using Random Forest Model
6 (2022)
16-27
Watershed , Terrain variables , Soil Properties , Random forest , Himalaya , Digital soil mapping
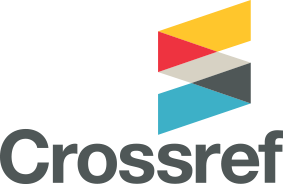

Soil information, including their spatial variability is vital for devising various soil and land management policies and strategies as well as assessing the environmental impact of different land use changes. Among the various soil forming factors, terrain plays a vital role in determining the spatial distribution of soil properties especially in hilly terrains due to its impact on soil processes as well as the redistribution of soil materials. The study was carried out for assessing the applicability and efficiency of various terrain parameters for prediction as well as spatial mapping of different soil properties using Random Forest (RF) modeling approach, in a mid-Himalayan watershed located in Tehri Garhwal district, Uttarakhand. Field data collection was done by collecting soil samples from 0-15cm depth (surface layer) at 68 different locations in the study area, following a transect survey method. Collected samples were pre-processed and analyzed in the laboratory for estimating various soil properties such as sand, silt and clay contents as well as soil nutrients such as Soil Organic Carbon (SOC) and nitrogen (N). Fifteen terrain parameters derived by digital terrain analysis of CartoDEM of 10m spatial resolution were used as predictor variables during RF modeling. Pearson correlation analysis revealed poor linear relationship of soil properties with various terrain parameters. Variable importance ranking revealed that compound topographic index, elevation, heat load index, integrated moisture index and mean curvature are the most important variables, explaining maximum variability in different properties. The performance of RF models for predicting various soil properties were evaluated based on the coefficient of determination (R2), Root Mean Square Error (RMSE) as well as Lin’s Concordance Correlation Coefficient (CCC). The models were found to perform well with R2 and CCC values of 0.47 and 0.50 for sand, 0.52 and 0.55 for silt, 0.44 and 0.57 for clay, 0.41 and 0.36 for organic carbon and 0.55 and 0.34 for nitrogen, respectively. The validated models were further employed for generating the spatial distribution maps of various soil properties. Such attempts combining various environmental variables with machine learning based models may aid us in mapping the spatial distribution of various soil properties in the hilly mountainous terrains with considerable accuracies.
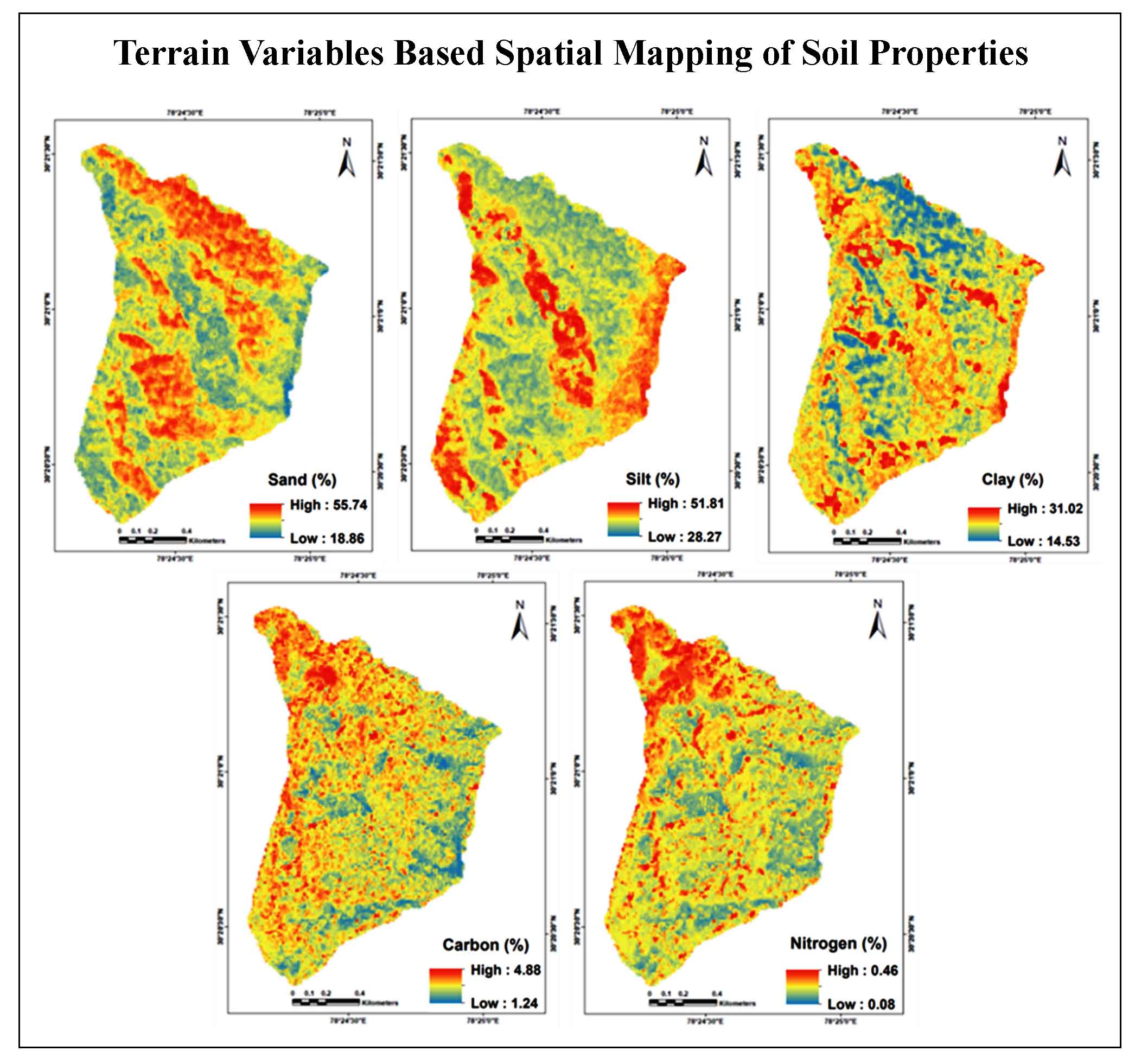
Terrain plays a vital role in determining the spatial distribution of soil properties in hilly terrains.
High resolution CartoDEM (10m resolution) used for generating different terrain variables.
Field collected soil samples used for generation of soil database and model development.
Random forest regression model employed for spatial prediction of soil properties using terrain parameters.
Compound topographic index, elevation, heat load index, integrated moisture index and mean curvature explain.
Validated models employed for mapping the spatial distribution of organic carbon, nitrogen and textural components.
Boettinger, J. L., Ramsey, R. D., Bodily, J. M., Cole, N. J., Kienast-Brown, S., Nield, S.J., Saunders, A.M. and Stum, A. K., 2008. Landsat spectral data for digital soil mapping. In: Hartemink, A.E., Mcbratney, A.B., Mendonça-Santos, M.L. (Eds.), Digital Soil Mapping with Limited Data. Springer-Verlag, New York, pp. 192-202.
Burrough, P. A., 1986. Principles of Geographic Information Systems for land resource assessment. Monographs on Soil and Resources Survey No. 12, Oxford Science Publications, New York.
Ciampalini, R., Martin, M., Saby, N., de Forges, A.C.R., Nehlig, P., Martelet, G. and Arrouays, D., 2014. Modelling soil particle-size distribution in the region “Centre” (France). In: Arrouays, D., McKenzie, N., Hempel, J., de Forges, A.C.R., McBratney, A. (Eds.), GlobalSoilMap: Basis of the Global Spatial Soil Information System. CRC Press, 325-331.
ESRI [Environmental Systems Research Institute], 2011. ArcGIS Desktop: Release 10. Redlands, CA.
Fu, P., and P. M. Rich. 2000. The Solar Analyst 1.0 Manual. Helios Environmental Modelling Institute (HEMI), USA.
Funnell, D. and Parish, R., 2005. Mountain Environments and Communities. Routledge, London.
Gastaldi, G., Minasny, B. and McBratney, A. B., 2012. Mapping the occurrence and thickness of soil horizons within soil profiles. In: Minasny, B., Malone, B.P., McBratney, A.B. (Eds.), Digital Soil Assessments and Beyond. CRC Press/Balkema, London, pp. 145–148.
Guo, P. T., Li, M. F., Luo, W., Tang, Q. F., Liu, Z. W. and Lin, Z. M., 2015. Digital mapping of soil organic matter for rubber plantation at regional scale: An application of random forest plus residuals kriging approach. Geoderma, 237, 49-59.
Lagacherie, P., McBratney, A. B. and Voltz, M., 2007. Digital SoilMapping: An Introductory Perspective. Elsevier, Amsterdem.
NRSC [National Remote Sensing Centre]. 2014. Evaluation of Indian National DEM (Version-2) from Cartosat-1 data. NRSC, ISRO.
Rich, P. M., Dubayah, R., Hetrick, W. A. and Saving, S. C., 1994. Using View shed Models to Calculate Intercepted Solar Radiation: Applications in Ecology. American Society for Photogrammetry and Remote Sensing Technical Papers, 524-529.